“Apple Inc. will allow its artificial intelligence teams to publish research papers for the first time”, reported Alex Webb of Bloomberg on Dec. 6, 2016, adding that the move “[marked] a significant change in strategy that could help accelerate the iPhone maker’s advances in deep learning.”
Apple’s initial announcement came as a surprise to many. The company had previously maintained staunchly insular policies regarding information pertaining to A.I tech development — and their progress within the field suffered as a result.
“There’s no way they can just observe and not be part of the community and take advantage of what is going on,” Yoshua Bengio, professor of Computer Science at the University of Montreal, told Bloomberg. “I believe if they don’t change their attitude, they will stay behind.”
Following the December 2016 announcement, Apple has demonstrated unprecedented transparency in its revitalized foray into the field of artificial intelligence, beginning with a research paper published Dec. 25, 2016.
“Six researchers from the company’s recently formed machine learning group published a paper that describes a novel method for simulated [and] unsupervised learning,” John Mannes, writer for TechCrunch, reported on Dec. 26, 2016. “The aim is to improve the quality of synthetic training images. The work is a sign of the company’s aspirations to become a more visible leader in the ever growing field of AI.”
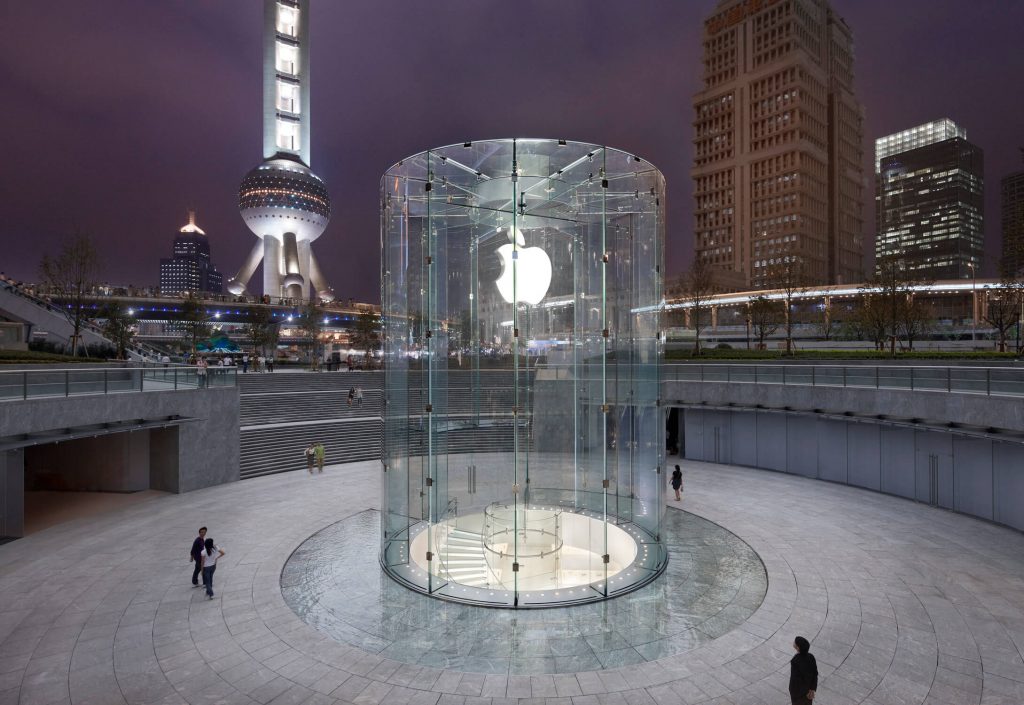
Machine learning, a form of computer science, allows for computers to react, adapt, learn, and make predictions based on data-driven algorithms instead of being ‘locked’ into following a set of programmed instructions. In a nutshell it allows the computer to think outside the box.
Various models of machine learning exist; more common applications include data-driven, informational models that operate at the heart of email spam filters, while other modes serve a fundamental function within many of our most utilized social media platforms, search engines, and more.
“Facebook’s News Feed uses machine learning to personalize each member’s feed”, writes Margaret Rouse of TechTarget. “If a member frequently stops scrolling in order to read or ‘like’ a particular friend’s posts, the News Feed will start to show more of that friend’s activity earlier in the feed.”
Put plainly it all boils down to pattern recognition and, subsequently, adaptation.
“Behind the scenes, the software is simply using statistical analysis and predictive analytics to identify patterns in the user’s data and use to patterns to populate the News Feed,” writes Rouse.
But Apple’s deep dive into the realm of machine learning suggests larger ambitions than social media analytics. While the company’s research is still in the early stages, it’s clear that Apple has stepped up to the plate in a major way.
On Jan. 27, 2017, Apple officially joined the Partnership on AI; a non-profit organization dedicated to researching the field of artificial intelligence. Other members of the group include fellow tech industry giants such as Facebook, Amazon, Google, IBM, and more.
More recently, Apple announced plans to expand upon its existing presence in Seattle’s Two Union Square tower, where the company already occupies two floors.
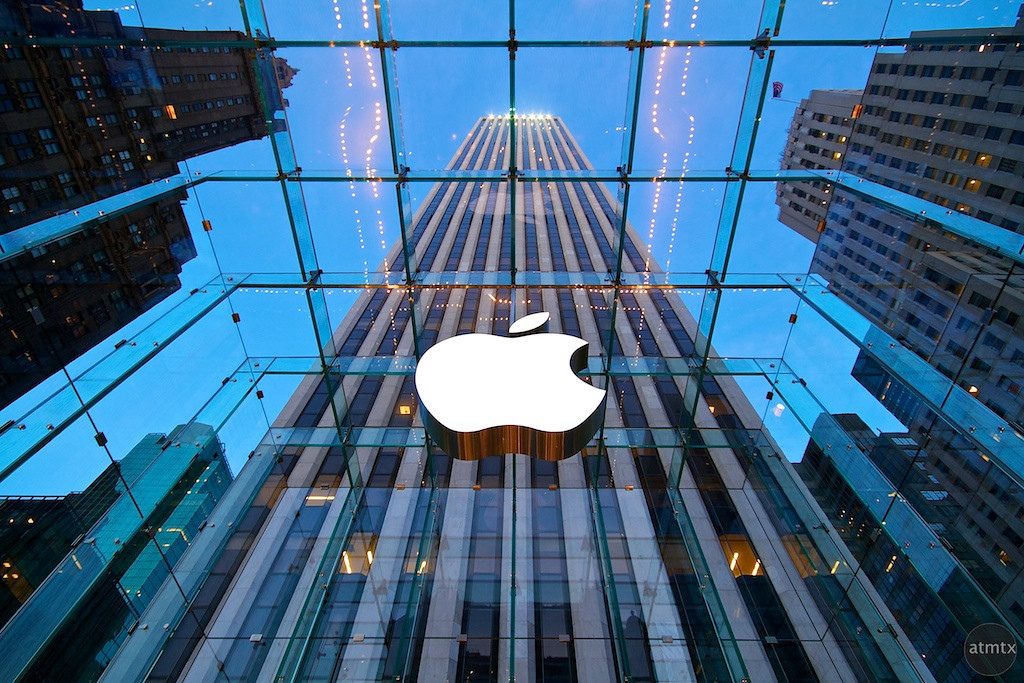
“In many ways, the tech world’s AI arms race is really a fight for talent. Skilled engineers are in short supply, and Silicon Valley’s biggest companies are competing to nab the best minds from academia and rival firms,” writes James Vincent of The Verge. “ Which is why it makes sense that Apple has announced it’s expanding its offices in Seattle, where much of its AI and machine learning work is done.”
In an interview with Geekwire, Carlos Guestrin, Apple’s director of machine learning, “…was ambiguous about Apple’s plans for AI and machine learning technology, but spoke about making people ‘emotionally connected’ to devices like iPhones through how much they understand their users and make relevant predictions,” Todd Bishop reports. “The Seattle team is said to be doing a mix of short- and long-term research, developing features for upcoming products as well as working on concepts that are 3 to 10 years out.”
The flurry of activity has demonstrated Apple’s newfound commitment to openness regarding its research, plans, and expansion; a demonstration that may very well attract top minds previously uninterested in partnering with the secretive corporation.
“The really strong people don’t want to go into a closed environment where it’s all secret,” Bengio said. “The differentiating factors are, ‘Who are you going to be working with?’ ‘Am I going to stay a part of the scientific community?’ ‘How much freedom will I have?’ ”
It would seem as though Apple has taken these questions to heart and adapted in kind.
“We’re trying to find the best people who are excited about AI and machine learning —excited about research and thinking long term but also bringing those ideas into products that impact and delight our customers,” Guestrin said.
A simulated, or “supervised”, model of machine learning relates to conceptual learning; the construction of key concepts and the subsequent categories by which to ‘sort’ and classify them. An unsupervised model, then, is more along the lines of improvisation.
In a supervised machine learning model, “the program is “trained” on a predefined set of “training examples”, which then facilitate its ability to reach an accurate conclusion when given new data”, writes Nick McCrea, software engineer at Toptal.
Conversely, in an unsupervised model, “the program is given a bunch of data and must find patterns and relationships therein”, writes McCrea.
The novelty behind Apple’s proposed approach comes from the marriage of these two modes of learning.
“In recent times, synthetic images and videos have been used with greater frequency to train machine learning models,” writes Mannes.
“Rather than use cost and time intensive real-world imagery, generated images are less costly, readily available and customizable. [But] it’s hard to ensure generated images meet the same quality standards as real images,” writes Mannes.
Apple’s research team is attempting to circumvent this issue through adopting a specific unsupervised mode of learning — generative adversarial networks, or GANS, which involve two competing neural networks. GANS pits one ‘generative’ network against one ‘discriminative’ network. Most frequently used to create photorealistic images, the generative network tries to ‘trick’ the discriminative network with sets of ‘true’ data and ‘generated’ data, while the discriminative network is responsible for validating or invalidating the ‘authenticity’ of the data sets. The end goal of this type of network is to have the generative network eventually fool the discriminative network with the generated data sets.
Imagine it this way; let’s say someone is attempting to sell an art collector a forgery. The first time around, the art collector dismisses the forgery, calling it out for being fake. But the forger is persistent; he keeps coming back with better forgeries, and eventually tricks the collector into purchasing a fake.